Multivariate Time Series Forecasting focuses on the prediction of future values based on historical context. State-of-the-art sequence-to-sequence models rely on neural attention between timesteps, which allows for temporal learning but fails to consider distinct spatial relationships between variables. In contrast, methods based on graph neural networks explicitly model variable relationships. However, these methods often rely on predefined graphs and perform separate spatial and temporal updates without establishing direct connections between each variable at every timestep. This paper addresses these problems by translating multivariate forecasting into a spatiotemporal sequence formulation where each Transformer input token represents the value of a single variable at a given time. Long-Range Transformers can then learn interactions between space, time, and value information jointly along this extended sequence. Our method, which we call Spacetimeformer, achieves competitive results on benchmarks from traffic forecasting to electricity demand and weather prediction while learning fully-connected spatiotemporal relationships purely from data.
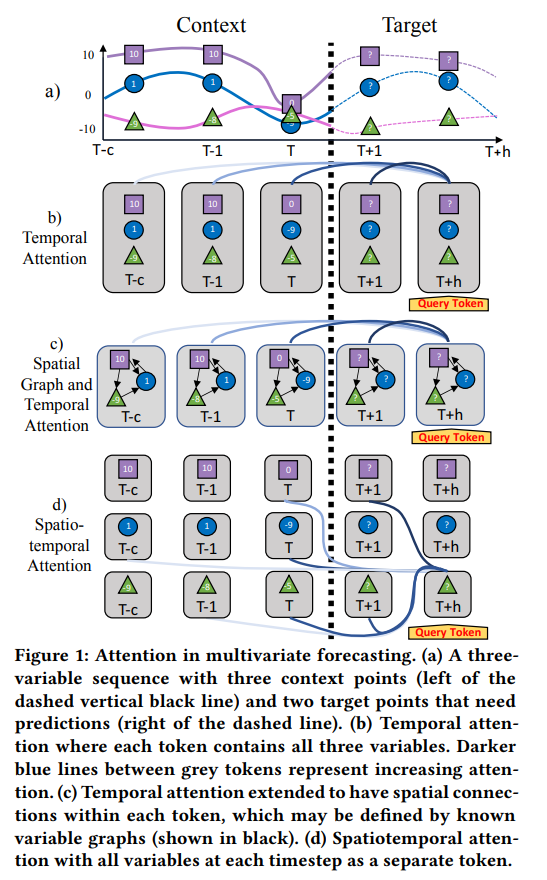
Fulldocs: https://towardsdatascience.com/multivariate-time-series-forecasting-with-transformers-384dc6ce989b
PDF: https://arxiv.org/pdf/2109.12218.pdf