Artificial intelligence powered by deep learning now solves challenges once thought impossible, such as computers understanding and conversing in natural speech and autonomous driving. Inspired by the effectiveness of deep learning to solve a great many challenges, the exponentially growing complexity of algorithms has resulted in a voracious appetite for faster computing. NVIDIA designed the Volta Tensor Core architecture to meet these needs.
NVIDIA and many other companies and researchers have been developing both computing hardware and software platforms to address this need. For instance, Google created their TPU (tensor processing unit) accelerators which have generated good performance on the limited number of neural networks that can run on TPUs.
In this blog, we share some of our recent advancements which deliver dramatic performance gains on GPUs to the AI community. We have achieved record-setting ResNet-50 performance for a single chip and single server with these improvements. Recently, fast.ai also announced their record-setting performance on a single cloud instance.
Our results demonstrate that:
- A single V100 Tensor Core GPU achieves 1,075 images/second when training ResNet-50, a 4x performance increase compared to the previous generation Pascal GPU.
- A single DGX-1 server powered by eight Tensor Core V100s achieves 7,850 images/second, almost 2x the 4,200 images/second from a year ago on the same system.
- A single AWS P3 cloud instance powered by eight Tensor Core V100s can train ResNet-50 in less than three hours, 3x faster than a TPU instance.
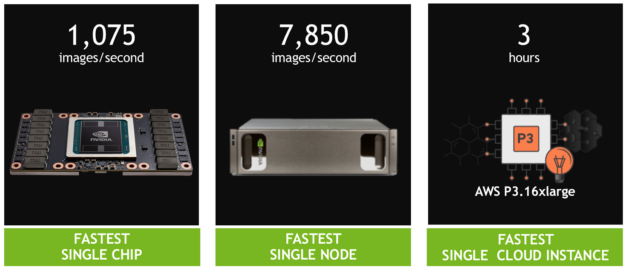
Massive parallel processing performance on a diversity of algorithms makes NVIDIA GPUs naturally great for deep learning. We didn’t stop there. Tapping our years of experience and close collaboration with AI researchers all over the world, we created a new architecture optimized for the many models of deep learning – the NVIDIA Tensor Core GPU.
Combined with high-speed NVLink interconnect plus deep optimizations within all current frameworks, we achieve state-of-the-art performance. NVIDIA CUDA GPU programmability ensures performance for the large diversity of modern networks, as well as provides a platform to bring up emerging frameworks and tomorrow’s deep network inventions …..
<read continue>